Ver ítem
- xmlui.general.dspace_homeCentros Regionales y EEAsCentro Regional Patagonia NorteEEA BarilocheArtículos científicosxmlui.ArtifactBrowser.ItemViewer.trail
- Inicio
- Centros Regionales y EEAs
- Centro Regional Patagonia Norte
- EEA Bariloche
- Artículos científicos
- Ver ítem
On the spatio-temporal coherence of extreme precipitation indices in subtropical Argentina
Resumen
This study evaluates the spatio-temporal coherence of regional extreme precipitation indices in subtropical Argentina (STAr) derived from rain gauge station data from 1991 to 2021. For the regionalization two machine learning clustering algorithms are used—Ward's method and K-means—and a novel stepwise regionalization approach, HAZ. While machine learning algorithms require the apriori definition of the optimal number of clusters, which varies
[ver mas...]
This study evaluates the spatio-temporal coherence of regional extreme precipitation indices in subtropical Argentina (STAr) derived from rain gauge station data from 1991 to 2021. For the regionalization two machine learning clustering algorithms are used—Ward's method and K-means—and a novel stepwise regionalization approach, HAZ. While machine learning algorithms require the apriori definition of the optimal number of clusters, which varies considerably with the used metric and selection criteria, the HAZ method relies on a Pearson's correlation coefficient threshold and avoids this limitation. In most cases machine learning algorithms struggled to produce coherent regions, with fewer clusters prioritizing spatial coherence at the expense of temporal consistency, and vice versa. Conversely, the HAZ method systematically outperformed machine learning approaches, providing regions with adequate spatio-temporal coherence. Notably, HAZ permits some stations to remain unclustered, allowing to reflect the local variability in extreme precipitation. The overall good performance of the HAZ method demonstrates its potential for broader applications in hydro-climatic studies. Moreover, two intensity indices were unsuitable for regionalization due to poor coherence, while the other three were prone to regionalization throughout the year. The Accumulated index, particularly using the 95th percentile as a threshold, emerged as the most representative, effectively synthesizing extreme precipitation characteristics in STAr. Finally, the necessity of validating the spatio-temporal internal coherence of clustering algorithms outputs is emphasized to avoid mischaracterization and ensure robust regionalization results.
[Cerrar]
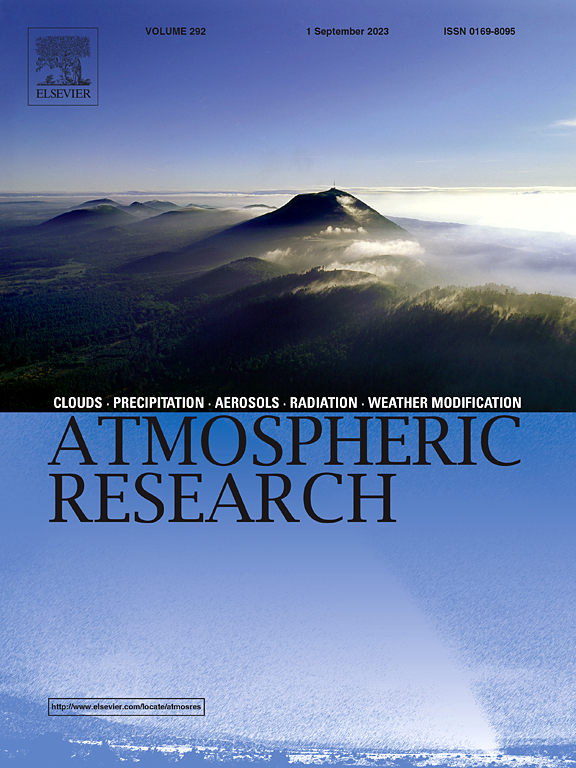
Autor
Ricetti, Lorenzo;
Hurtado, Santiago Ignacio;
Agosta Scarel, Eduardo A.;
Fuente
Atmospheric Research 320 : 108082 (July 2025)
Fecha
2025-07
Editorial
Elsevier
ISSN
0169-8095
1873-2895
1873-2895
Documentos Relacionados
Formato
pdf
Tipo de documento
artículo
Proyectos
(ver más)
INTA/2023-PD-L02-I091, Adaptación a la variabilidad y al cambio global: herramientas para la gestión de riesgos, la reducción de impactos y el aumento de la resiliencia de socioecosistemas
Palabras Claves
Derechos de acceso
Restringido
