Ver ítem
- xmlui.general.dspace_homeCentros Regionales y EEAsCentro Regional Patagonia NorteEEA BarilocheArtículos científicosxmlui.ArtifactBrowser.ItemViewer.trail
- Inicio
- Centros Regionales y EEAs
- Centro Regional Patagonia Norte
- EEA Bariloche
- Artículos científicos
- Ver ítem
Assessing Sirex noctilio Fabricius (Hymenoptera: Siricidae) Damage in Pine Plantations Using Remote Sensing and Predictive Machine Learning Models
Resumen
Early detection and monitoring of invasive forest pests are crucial for effective pest management, particularly in preventing large-scale damage, reducing eradication costs, and improving overall control effectiveness. This study investigates the potential of machine learning models and remote sensing at various spatiotemporal scales to assess forest damage caused by the woodwasp Sirex noctilio in pine plantations. A Random Forest (RF) model was applied
[ver mas...]
Early detection and monitoring of invasive forest pests are crucial for effective pest management, particularly in preventing large-scale damage, reducing eradication costs, and improving overall control effectiveness. This study investigates the potential of machine learning models and remote sensing at various spatiotemporal scales to assess forest damage caused by the woodwasp Sirex noctilio in pine plantations. A Random Forest (RF) model was applied to analyze Planetscope satellite images of Sirex-affected areas in Neuquén, Argentina. The model’s results were validated through accuracy analysis and the Kappa method to ensure robustness. Our findings demonstrate that the RF model accurately classified Sirex damage levels, with classification accuracy improving progressively over time (overall accuracy of 87% for five severity categories and 98% for two severity categories). This allowed for a clearer distinction between healthy and Sirex-infested trees, as well as a more refined categorization of damage severity. This study highlights the potential of machine learning models to accurately assess tree health and quantify pest damage in plantation forests, offering valuable tools for large-scale pest monitoring.
[Cerrar]
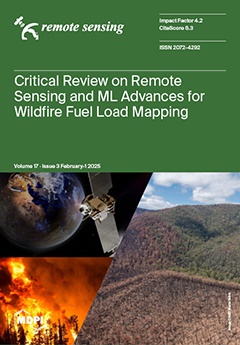
Autor
Hirigoyen, Andrés;
Villacide, Jose Maria;
Fuente
Remote Sensing 17 (3) : 537 (February 2025)
Fecha
2025-02
Editorial
MDPI
ISSN
2072-4292
Documentos Relacionados
Formato
pdf
Tipo de documento
artículo
Proyectos
(ver más)
INTA/2023-PD-L01-I074, Bases ecológicas y epidemiológicas para el diseño de estrategias de manejo de plagas agrícolas y forestales
INTA/2023-PE-L03-I033, Gestión Sostenible de los sistemas forestales naturales y cultivados para el desarrollo de los territorios y la provisión de servicios ecosistémicos en Patagonia Andina
Palabras Claves
Derechos de acceso
Abierto
